Causation
The correlation coefficient measures the strength of a linear relationship between two variables. Thus, it makes no implication about cause or effect. The fact that two variables tend to increase or decrease together does not mean that a change in one is causing a change in the other.
At times, two variables may be strongly correlated because they are equally correlated to a third (either known or unknown) variable. Such variables are called lurking variables. Lets take the following example:
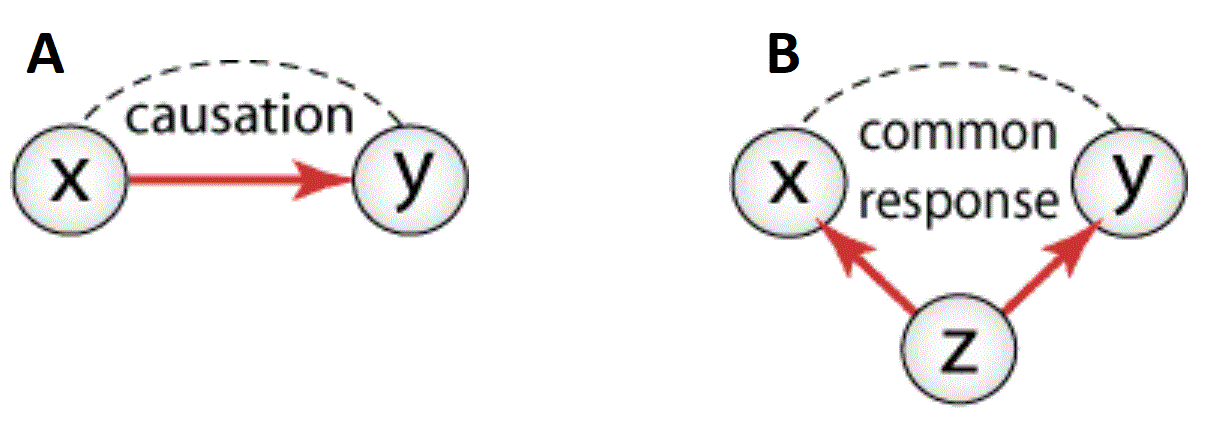
Figure 6.8: Examples of lurking variables
In the figure above, the dashed lines show an association. The solid red arrows show a cause-and-effect link. The variable x is explanatory, y is a response variable, and z is a lurking variable.
Basically, in example B, you will find a strong correlation between x and y, not because they are causality related, but because they are both strongly affected by a variable you did not measure (i.e., Z in the case example above) or a so-call lurking variable. The effect is that you can make a spurious conclusion if you interpret the coefficient correlation as a cause and effect.
For instance, you know that in tropical countries there is a strong correlation between the consumption of ice cream and shark attacks?. One could conclude that sharks like sweet people?. Hmm…think about this correlation….is there a lurking variable here?. what could it be?
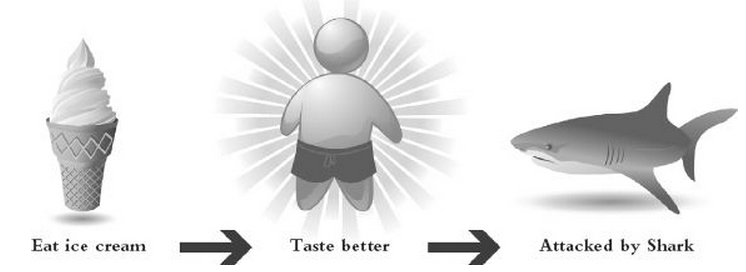
Figure 6.9: Example of a lurking variable